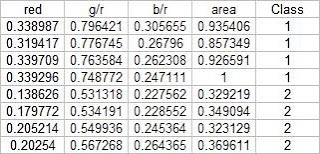
Using the above training set and the methods described in the provided pdf file, we can compute for the covariance matrix given below:
0.0062310 0.0087568 0.0012735 0.0226916
0.0087568 0.0129916 0.0019430 0.0329768
0.0012735 0.0019430 0.0005591 0.0042263
0.0226916 0.0329768 0.0042263 0.0876073
The test set is given below:
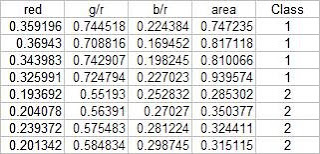
From this test set, the computed discrimant values are:
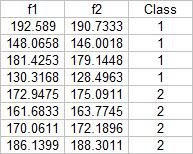
-----------------------------------------------------------------------
chdir("C:\Users\RAFAEL JACULBIA\Documents\subjects\186\activity19");
x = [];
y = [];
x1 = [];
x2 = [];
u1 = [];
u2 = [];
x1o = [];
x2o = [];
c1 = [];
c2 = [];
C = [];
n = 8;
n1 = 4;
n2 = 4;
x = fscanfMat("x.txt");
y = fscanfMat("y.txt");
test = fscanfMat("test.txt");
x1 = x(1:n1,:);
x2 = x(n1+1:n,:);
u1 = mean(x1,'r');
u2 = mean(x2,'r');
u = mean(x,'r');
x1o(:,1) = x1(:,1) - u(:,1);
x1o(:,2) = x1(:,2) - u(:,2);
x1o(:,3) = x1(:,3) - u(:,3);
x1o(:,4) = x1(:,4) - u(:,4);
x2o(:,1) = x2(:,1) - u(:,1);
x2o(:,2) = x2(:,2) - u(:,2);
x2o(:,3) = x2(:,3) - u(:,3);
x2o(:,4) = x2(:,4) - u(:,4);
c1 = (x1o'*x1o)/n1;
c2 = (x2o'*x2o)/n2;
C = (n1/n)*c1 + (n2/n)*c2;
p = [n1/n;n2/n];
class = [];
for i = 1:size(test,1)
f1(i) = u1*inv(C)*test(i,:)'-0.5*u1*inv(C)*u1'+log(p(1));
f2(i) = u2*inv(C)*test(i,:)'-0.5*u2*inv(C)*u2'+log(p(2));
end
f1-f2
------------------------------------------------------------------------
I proudly grade myself 10/10 for getting it perfectly without the help of others. I also consider this activity one of the easiest because of the step by step method given in the provided file.
No comments:
Post a Comment