OBJECT
Two methods are used in this activity, non-parametric and parametric. Parametric segmentation is done by determining the probability that a region belongs to the region of interest. In particular, a gaussian probability distribution function is assumed. For non-parametric segmentation, the frequency value of the pixel in the histogram itself is used to backproject the value for that pixel. The two dimensional histogram of the original image test image is shown below:
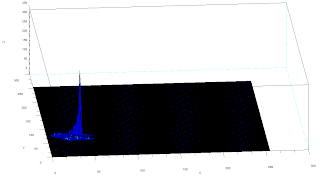
The following are the results of the color segmentation:
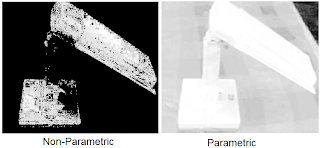
----------------------------------------------------------------------------------------------
----------------------------------------------------------------------------------------------
Scilab Codes:
Parametric
I = imread("lamp_section.jpg");
orig = imread("lamp_orig.jpg");
av = I(:,:,1)+I(:,:,2)+I(:,:,3);
r = I(:,:,1)./av;
g = I(:,:,2)./av;
b = I(:,:,3)./av;
av2 = orig(:,:,1)+orig(:,:,2)+orig(:,:,3);
r2 = orig(:,:,1)./av2;
g2 = orig(:,:,2)./av2;
b2 = orig(:,:,3)./av2;
r = r*255;
g = g*255;
frequency = zeros(256,256);
sizer = size(r);
for i=1:sizer(1)
for j=1:sizer(2)
x = abs(round(r(i,j)))+1;
y = abs(round(g(i,j)))+1;
frequency(x,y) = frequency(x,y)+1;
end
end
//imshow(log(frequency+0.0000000001));
r = r/255;
g = r/255;
mr = mean(r);
devr = stdev(r);
mg = mean(g);
devg = stdev(g);
pr = (1/(devr*sqrt(2*%pi)))*exp(-((r2-mr).^2)/2*devr);
pg = (1/(devg*sqrt(2*%pi)))*exp(-((g2-mg).^2)/2*devg);
prob = pr.*pg;
prob = prob/max(prob);
scf(0);imshow(orig);
scf(1);imshow(prob,[]);
-------------------------------------------------------------------------------------------------
Non Parametric:
I = imread("lamp_section.jpg");
orig = imread("lamp_orig.jpg");
sizeO = size(orig)
av = I(:,:,1)+I(:,:,2)+I(:,:,3);
r = I(:,:,1)./av;
g = I(:,:,2)./av;
b = I(:,:,3)./av;
av2 = orig(:,:,1)+orig(:,:,2)+orig(:,:,3);
r2 = orig(:,:,1)./av2;
g2 = orig(:,:,2)./av2;
b2 = orig(:,:,3)./av2;
r = r*255;
g = g*255;
frequency = zeros(256,256);
sizer = size(r);
for i=1:sizer(1)
for j=1:sizer(2)
x = abs(round(r(i,j)))+1;
y = round(g(i,j))+1;
frequency(x,y) = frequency(x,y)+1;
end
end
scf(0);imshow(log(frequency+0.0000000001));
r2 = r2*255;
g2 = g2*255;
seg = zeros(sizeO(1),sizeO(2));
sizer2 = size(r2);
for i = 1:sizer2(1)
for j = 1:sizer2(2)
x = abs(round(r2(i,j)))+1;
y = round(g2(i,j))+1;
seg(i,j) = frequency(x,y);
end
end
scf(1);imshow(log(seg+0.000000000001),[]);
scf(2);imshow(orig);
-------------------------------------------------------------------------------------------------
-------------------------------------------------------------------------------------------------
I will give myself a grade of 10 for this activity since the objectives were met and the results are interesting.
Collaborator:
Ed David


No comments:
Post a Comment